Henry Kissinger
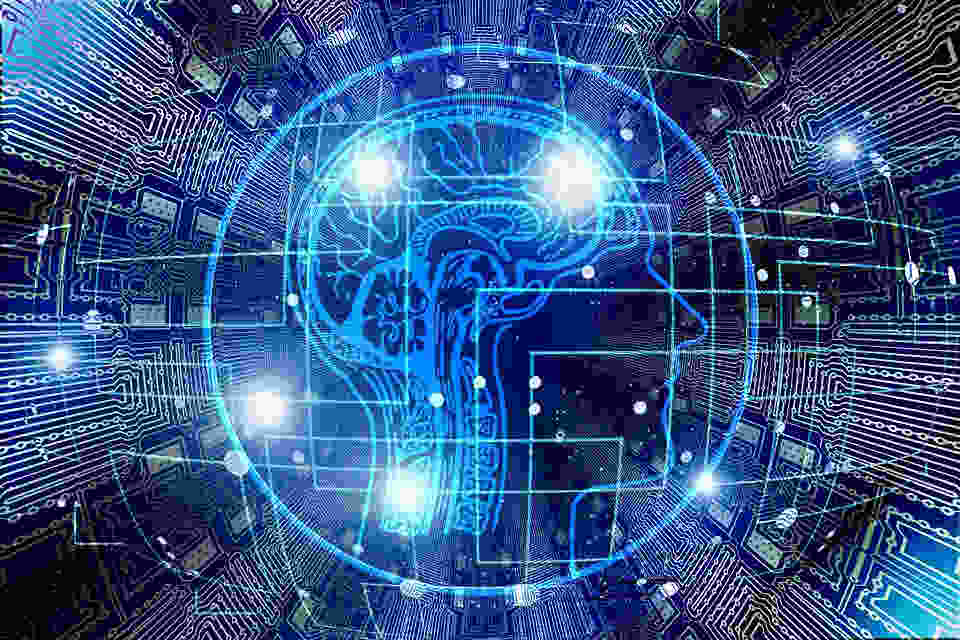
A Historical Perspective
Let’s take a look at how we got here and why AI is suddenly capturing so much attention. We will revisit a little bit of the history of AI and the convergence of three growth vectors: Algorithmic advances, computing power, and data explosion. These vectors have their own historical landmarks as outlined below, until they converge around 2007, when the iPhone was first introduced.
The first vector, algorithmic advances, goes back as far as 1805 when French mathematician Adrien-Marie Legendre published the least square method of regression which provides the basis for many of today’s machine-learning models. In 1965 the architecture for machine deep-learning using artificial neural networks was first developed. Between 1986 and 1998 we saw a number of algorithmic advances: backpropagation, which allows for optimization without human intervention; image recognition; natural language processing; and Google’s famous PageRank algorithm.
The second vector, computing power, had a significant historical landmark in 1965 when Intel cofounder Gordon Moore recognized the exponential growth in chip power: the number of transistors per square inch doubles every year. This became known as Moore’s law, and has correctly predicted the doubling of computing power every 18 months to the present day and into the foreseeable future. At the time the state-of-the-art computer was capable of processing in the order of 3 million FLOPS (floating-point operations per second). By 1997, IBM’s Deep Blue achieved 11 giga FLOPs (11 billion FLOPS), which led to its victory over Gary Kasparov, the world chess champion. In 1999 the Graphics Processing Unit (GPU) was unveiled – a fundamental computing capability for deep learning. In 2002 we saw the advent of Amazon’s Web Services (AWS) making computing power easily available and affordable through cloud computing. In 2004 Google launched MapReduce, which allows computer to deal with immense amounts of data by using parallel processing, leading to the introduction of Hadoop in 2006, which allowed companies to deal with the avalanche of data produced by the web.
Finally, the third vector, data explosion, started is 1991 when the world wide web was made available to the public. In the early 2000’s we saw wide adoption of broadband, which opened the doors to many internet innovations, resulting in the debut of Facebook in 2004, and Youtube in 2005. At this time, the number of internet users worldwide surpassed one billion.
The year 2007 became a significant landmark. It is at this point that the technologies begin converging and intercepting new horizons as the mobile explosion came to life with Steve Job’s announcement of the iPhone in 2007. From here several significant advances give birth to a renewed enthusiasm for Artificial Intelligence. In 2009, Stanford University scientists showed they could train deep-belief networks with 100 million parameters using GPUs at a rate 70 times faster than using CPUs. By 2010, 300 million smartphones were sold, and internet traffic reached 20 exabytes (20 billion gigabytes) per month.
In 2011 a key milestone was achieved. IBM Watson defeated the two greatest Jeopardy champions, Brad Ruttner and Ken Jennings. Such achievement was made possible by IBM servers capable of processing 80 teraFLOPS (80 trillion FLOPS). Remember that when Moore’s law was pronounced in the mid 60’s, the most powerful computer could only process 3 million FLOPS.
By 2012 significant progress had been made in deep learning for image recognition. Google used 16,000 processors to train a deep artificial neural network to recognize images of cats in YouTube videos without providing any information to these machines about the images. Convolutional Neural Networks (CNN) became capable of classifying images with a high degree of accuracy. In the meantime, the data explosion continued, with the number of mobile devices on the planet exceeding the number of humans, generating 2.5 quintillion bytes of data per day by 2017. Computing power reached new heights as Google announced its Tensor Processing Units (TPU) capable of 180 million teraFLOPS.
It is at this point in the history of Artificial Intelligence that many people started to realize we might not be too far from achieving, or even exceeding, what is known as Artificial General Intelligence (AGI). To the astonishment of the world, Google’s DeepMind hit another major milestone when its AlphaZero algorithm learned to play by itself the games of chess, shogi and Go (Go is a very complex game, much more challenging than chess). Not only did AlphaZero learn to play by itself, it defeated the best computers that had been fed instructions from human experts! And it did this in only eight hours of self-play!
AlphaZero, by itself, came up with playing strategies that humans had never thought of before – that is a significant event that would explain why there is so much buzz about AI at this point in time.
Human vs. Machine Intelligence Milestones


Has Human Intelligence Already Been Defeated by Machines?
Looking at these milestones, you can’t help but wonder if human intelligence has already been defeated my machine intelligence. The answer is no. There are specific areas in which machines seem to have defeated the human brain, but there is much more to human-level intelligence than the narrow applications in which human brains have been defeated. The level of complexity and capability of the human mind is nowhere near being matched by computers – at least not yet.
The human brain is an amazing, wondrous and mysterious creation. Princeton researchers have shown that humans make judgements about other humans within 100 milliseconds of meeting them. Through a process that scientists call thin slicing, humans make judgmental decisions about others such as whether they are trustworthy, smart, high-status, gay or straight, successful, adventurous, and more. All in less than a second.
Even a three-year-old child is capable of making visual identifications that today’s most sophisticated super-powered computer algorithms are nowhere near capable of matching.
The (small) Elephant in the Room
To prove the point, a new study found that artificial intelligence systems fail a vision test that a child could accomplish with ease. The study presented an artificial intelligent system a living room scene which the system processed well, recognizing images such as a chair, a couch, a TV, etc. But when the scientists introduced an anomalous object into the scene – the image of an elephant, the system became very ‘confused’ and made several mistakes, identifying a chair as a couch, and completely missing other objects that it had identified before.
“There are all sorts of weird things happening that show how brittle current object detection systems are,” said Amir Rosenfeld, a researcher at York University in Toronto and co-author of the study along with his York colleague John Tsotsos and Richard Zemel of the University of Toronto.
The problem is due to the laborious ways in which Artificial Intelligence processes visual impressions, one layer at a time. Contrast this to how the human brain takes in a staggering amount of information at once and processes it instantaneously. “We open our eyes and everything happens,” said Tsotsos.
Critics of the ‘over-hyping’ of Artificial Intelligence are quick to point out these flaws and many others. They pronounce that the AI revolution hasn’t happened yet. They remind us that the term Artificial Intelligence as it was coined in the late 1950’s to refer to the heady aspiration of realizing in software and hardware an entity possessing human-level intelligence, should not be used interchangeably with Machine Learning. Machine Learning is an algorithmic field that blends ideas from statistics, computer science and other disciplines to process data, make predictions and help make decisions – but it does equate to human-level intelligence.
It is clear that we may be misusing the term Artificial Intelligence as it was originally intended and that we may be applying it too broadly to refer to much less capable technologies. It is also clear that machines are nowhere near the level of capability of the human brain.
However, we can’t ignore the astonishing rate of improvement observed in the last few years. Most scientists believe that the Artificial Intelligence challenges faced today will be conquered sooner than we suspect. In fact, most experts agree that we will achieve human-level artificial intelligence this century, many believing it will happen as soon as in the next 20 to 40 years.
Intelligence Explosion
In order to understand the challenges that lay ahead, we must first look at the different categories of Artificial Intelligence:
1) Artificial Narrow Intelligence (ANI) – You can think of ANI as a specialized intelligence. For example, the algorithms of a search engine, natural language processing, self-driving cars, Siri, or even the self-learning machines that mastered the game of Go.
2) Artificial General Intelligence (AGI) – This refers to the human-level intelligence that the original Artificial Intelligence term was coined after. Creating AGI is a much harder task than creating ANI, and we are, at this point in time, not even close to achieving it. This level of intelligence requires a very general cognitive capability that, among other things, involves the ability to reason, plan, solve problems, think abstractly, comprehend complex ideas, learn quickly, and learn from experience.
3) Artificial Superintelligence (ASI) – Nick Bostrom, author of the book Superintelligence: Paths, Dangers, Strategies, defines superintelligence as “an intellect that is much smarter than the best human brains in practically every field, including scientific creativity, general wisdom and social skills.” An ASI agent could be trillions of times smarter than human beings. That is where things get very scary. To make matters worse, once AGI is achieved, ASI may not be too far behind.
As we have exemplified above, ANI is already here. AGI, however, is still just a sparkle in a scientist’s eye. As we have seen in the historical progression of Artificial Intelligence, computational power has always been a pre-requisite to the next level of progress. Getting to AGI is no different. To give you a sense of the current state of affairs, scientists have estimated that the human brain is capable of approximately 10 quadrillion computations per second (cps). The world’s fastest computer, the Tianhe-2 from China, has already beaten this number, as it can do about 34 quadrillion cps. However, the Tianhe-2 is a huge beast that costs $390 million to build.
Ray Kurzweil, a futurist who is very optimistic about Artificial Intelligence, estimates that AGI can become widespread when we can get 10 quadrillion cps for about $1,000. Using Moore’s law which, as we have seen, has correctly predicted the growth of computing power so far, it is estimated that we will be there by 2025, only 7 years away.
But computing power is only one prerequisite. In order to achieve AGI, we still need to figure out how to create human-level intelligence. How are going to get there? Scientists are working on reverse engineering the human brain. The techniques have already been developed. In fact, we are able to emulate a 1 mm long flatworm brain with 302 neurons today. Now we just need to figure out how to scale this technique to 100 billion neurons, the approximate size of the human brain. There are optimistic estimates that we will achieve this by 2030.
There are also efforts to build computers that specialize in AI research. That means that the computer themselves will become smarter at each iteration of progress, until AGI is achieved. This could happen very soon. Remember that computers can operate at exponential growth, something that it is hard for us humans to understand. What appears to be progressing very slowly could suddenly explode into reality.
Once we reach AGI, given the same iterative progression on an exponential curve, it is not hard to foresee that ASI will arrive shortly after. The intelligence explosion will then reach a level that we can’t even comprehend – and therein lies the dangers.
Humans have dominated the earth due to our intelligence. It is easy for us to see that with intelligence comes power. Once we create an ASI agent that is vastly more intelligent than we are, what will be our fate?
This is a very difficult question to ponder. Let’s look at ways in which we may try to deal with Superintelligence and influence its outcomes.
Living with Superintelligence
If the advent of Superintelligence is inevitable, can we control it so that it will behave in ways that are beneficial and not harmful to humanity?
Nick Bostrom, in his book Superintelligence: Paths Dangers Strategies, describes several control methods, all of which have their own sets of potential benefits and shortcomings.
Boxing – This may be one of the most obvious control mechanisms that comes to mind. You put the ASI agent in a confinement where contact with the external world is restricted by humans. If it misbehaves you shut it down. Can we expect that this Superintelligent agent won’t outsmart us in finding a way to escape if it is motivated to do so?
Incentive Methods – We could build an ASI in such a way that its behaviors are controlled by rules, or incentive mechanisms. However, if the ASI obtains a ‘decisive strategic advantage’, legal and economic incentives and sanctions may not be sufficient to influence its behavior. The ASI may internalize and develop its own norms and ideologies and regard these human-made rules as meaningless.
Stunting – This is a method in which you impose constraints on the ASI’s cognitive capabilities. This approach has three fundamental flaws: First, limiting the capabilities of an algorithm would be counter-productive; Second, it is unlikely that we are capable of resisting the motives to build fully capable ASIs; Third, how do we define the threshold of cognitive capability and eliminate human biases? Set it incorrectly and it may figure out by itself how to become more intelligent and bypass the limitations imposed by humans.
Tripwires – This involves establishing a set of tests, potentially without the ASI’s knowledge, to detect any negative behavior or intent, and upon detection shut it down. You could, for instance, try to detect if the ASI contained in a box is trying to escape by establishing an internet connection. The problem here goes back to the fact that you are dealing with a Superintelligent agent who could easily subvert any tripwire devised by the human intellect.
Control methods, as explained above, may have limited effectiveness in preventing ASIs from behaving in undesirable ways. But what if we could take a different approach to the problem and try to prevent undesirable outcomes by shaping what the ASIs want to do? This is called Motivation Selection.
There are different approaches within Motivation Selection, as described below. Again, they have their own sets of potential benefits and shortcomings:
Direct Specification – This approach tries to explicitly define a set of rules or values that will be used as a compass by the ASI. This may seem straightforward, until you consider what set of rules and values we would wish the ASI to be guided by. And even if we got that perfectly right (which is highly unlikely) how would we go about expressing those rules and values in computer-readable code?
Domesticity – This approach is designed to severely limit the scope of the ASI’s ambitions and activities. Here we would have to define what appropriate limitations to use, so that the ASI would still be useful, but only to the extent that it minimizes its impact on the world. Again, we run into the same issue raised with the stunting control method of how to resist the motives to create a more powerful ASI.
Indirect Normativity – This is an interesting approach, but as we will see later, raises many ethical questions. The idea here is that instead of specifying the rules that guide the ASI’s behavior directly, you define the process by which the rules will be derived. In other words, instead of telling the ASI what to do, tell it to go figure out what we would have told it to do had we known better, and implement that. We will discuss this further when we review the idea of ‘extrapolating our volition’.
Augmentation – In this approach, instead of developing a new motivation system, we start with an existing one and enhance its cognitive abilities to make it Superintelligent. We are talking about brain emulations and biological enhancements. Do we, humans, have the right motivation systems to begin with? Even if the answer is positive, what prevents it from becoming corrupt as it gets enhanced? And do we really want to live in a world where ‘brains in a box’ with full human consciousness are copied, manipulated and discarded for scientific purposes?
If you haven’t realized yet the depth of the ethical dilemmas that are about to hit us in the next few decades, you may start to get a sense for it now. In addition to confronting the perplexing complications of manipulating the brain while preserving human dignity, we are starting to question entire value and motivation systems.
The (BIG) Elephant in The Room
As discussed, methods to control the behavior of an ASI are limited in effectiveness. The most promising approach appears to be using Motivation Selection to design an ASI that is seeded with the ‘right’ values to being with. But what are the ‘right’ values? And who decides?
If we, with all of our flaws, are incapable of seeding the values that will drive the behavior of a more intelligent being, should we leave it up to this superior being to determine its own set of values to drive our behaviors?
Eliezer Yudkowsky, an American Researcher who is popularizing the idea of a friendly AI, has proposed seeding the AI with what he calls our ‘Coherent Extrapolation Volition’ (CEV). Here is how CEV is defined by Yudkowsky:
“Our coherent extrapolation volition is our wish if we knew more, thought faster, were more the people we wish we were, had grown up farther together; where the extrapolation converges rather than diverges, where our wishes cohere rather than interfere; extrapolated as we wish that extrapolated, interpreted as we wish that interpreted.”
Dissecting the meaning of CEV is beyond the scope of this article. However, what Yudkowsky is trying to do is to come up with a morality model that encapsulates moral growth but keeps humanity ultimately in charge of its own destiny.
There are other morality models that are proposed, but the fundamental question is this: What moral values do we want to seed the AI with? If we don’t know, are we comfortable letting an all-powerful algorithm determine the moral values that will guide humanity for the rest of its existence?
Conclusion
We have some really big and hairy issues in front of us. We don’t know how we will address these difficult questions, but the time to start these conversations is now. As we pointed out earlier, AGI, and shortly after, ASI, is likely going to be a major part of our reality in a short few decades.
How many congressmen do you know who have a good grasp of the issues above? How many CEOs are thinking about how values guide the decisions of their organizations, and how those values might influence the behavior of machine Superintelligence?
If we don’t start addressing these issues now, we may run out of time. The consequences are unfathomable.
No comments:
Post a Comment