SYDNEY J. FREEDBERG JR.
WASHINGTON: Having bet heavily on artificial intelligence, the Pentagon now has a two-pronged plan to overcome the biggest obstacle to AI: the data.
As the short-term solution, the newly created Joint Artificial Intelligence Center, JAIC, will seek to gather the vast amounts of data required to train machine learning algorithms.
At the same time, DARPA will pursue the long-term goal of developing a next-generation artificial intelligence that’s intelligent enough to reach conclusions from less data.
Both tasks are daunting. Right from the start, “as we roll out the first two, three, four applications, the thing that will be hitting us over and over again will be data,” Dana Deasy, the Pentagon’s Chief Information Officer and chief overseer of JAIC, told the House Armed Services Committee on Tuesday. “Do we really understand where the sources of our data come from?…. How do you ingest it, what are its formats, do we have duplicative data, and how do we bring it together?”
“We’re partnering with the (Pentagon) CMO and the chief data management officer to start to identify what are going to be those problematic data sets where we’re going to have to get clearer standards,” Deasy said.
In the longer term, “we have to be smarter about how we understand how these algorithms are working,” said Lisa Porter, deputy undersecretary for research & engineering. Led by undersecretary Mike Griffin, Porter’s part of the Pentagon oversees both DARPA — which funds high-risk, high-payoff research — and the Defense Innovation Unit (formerly DIUx) — which looks for private-sector innovations that are already ready to try out.
“It’s not going to be just about , ’how do we get a lot of data?’” Porter told the House subcommittee. “It’s going to be about, how do we develop algorithms that don’t need as much data? How do we develop algorithms that we trust?”
Lisa Porter
All this will take a major scientific effort. Is the US up to it, HASC emerging threats subcommittee chairwoman Elise Stefanek asked, or “are we falling behind already?”
“We are not behind,” Porter said. “Right now, we are actually ahead. However, we are in danger of losing that leadership position.” The US and its allies — notably Britain and Canada — have an unmatched depth of academic talent, she said, in part because DARPA has been funding AI research for decades. But China, she said, is now investing massively in a talent pool of its own.
JAIC: Marshaling The Data
Machine learning requires a lot of data, but there’s a lot of data out there. The Pentagon’s problem is getting access to it.
Even within the Department of Defense, countless bureaucratic fiefdoms have their own databases with their own standards that are often incompatible with anyone else’s. Beyond DoD, there’s even more data available either for free or for sale from private companies and universities — if they’re willing to work with the military, and if the military’s byzantine contracting and classification rules don’t get in the way. Bringing all this data together so AI developers can access it will require both technological and institutional change.
Technologically, Pentagon CIO Deasy is counting on DoD’s move to cloud computing to connect the pockets of data: AI and the cloud are “mutually reinforcing, mutually dependent,” he told the House subcommittee. Instead of every military base or Pentagon office having their data on their own server in some closet, wired to their own desktop computers and nothing else, everyone’s data will reside on the same centralized server farms, with local users downloading what they need as they need it. (That said, he noted, users in war zones and other harsh environments will need to store more data locally — on the “edge” — to guard against losing access).
Of course, just because your data and my data are stored on the same machine doesn’t mean they’re in compatible formats: It’s like a library where books in English, Russia, Dutch, and ancient Greek are all shelved together. But at least Deasy & co. will know where it all is, and roughly what it is, so they can start converting it to common standards — and require all future data collection to conform to common standards from the start. That will also require establishing “very clear rules of the road” on who owns the data going forward, Deasy testified.
The Joint Artificial Intelligence Center will be at the center of the effort, Deasy said. Officially announced just in June, JAIC now has 30 full-time staff — a mix of military officers and civil servants from all over the Defense Department — and is recruiting more, including from universities and tech companies. (This will require changing the usual bureaucratic process for hiring and paying people, Deasy warned). In November, JAIC held its first-ever industry day with over 600 attendees from some 380 companies and academic institutions, many of which not only attended the general briefings but did private presentations of their technology to Pentagon officials.
“They are not just looking to traditional defense contractors,” said Graham Gilmer, a Booz Allen Hamilton executive who attended the industry day. “They are not just looking to major tech companies (or even) academia… They even made reference to one-person startups.”
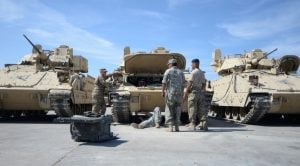
What will this actually produce for the ordinary soldier? No Terminators here: Deasy said the two near-term applications will be disaster relief — applying mapping and search techniques from the infamous Project Maven to saving lives — and predictive maintenance, which uses AI to determine the warning signs of, say, an engine breaking down so human mechanics can fix it beforehand.
For example, Booz Allen Hamilton is developing a predictive maintenance smartphone app. You download the app, then hold your phone up to a diesel generator, BAH’s Gilmer told me; the app will analyze the noises the generator’s making and tell you if it’s running properly or needs repair. It’s like the old guy at the garage who can figure out what’s wrong with your car just by listening to the engine, only now every 18-year-old private can do it with their phone.
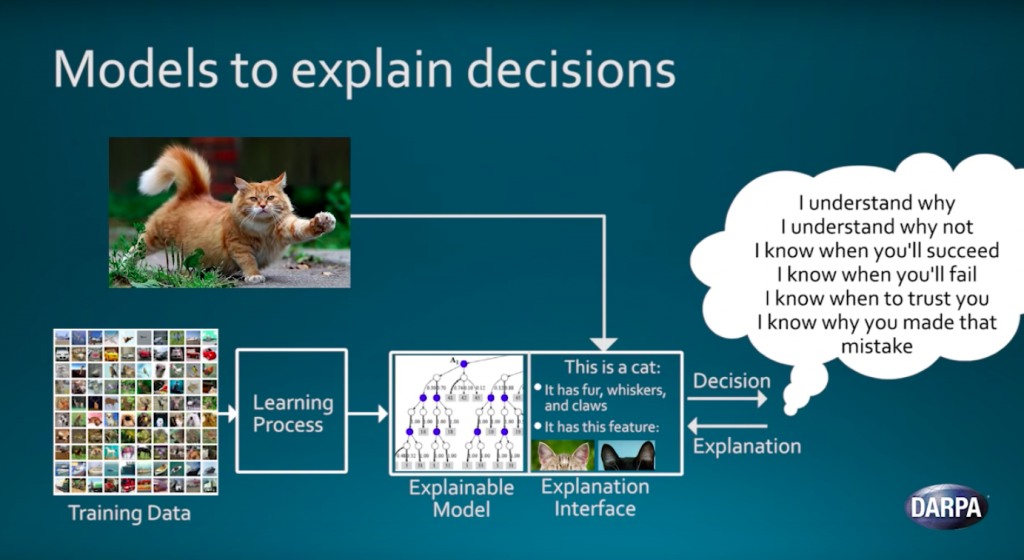
DARPA’s Next AI: As Smart As A Toddler?
For all the wonders of GPS navigation and voice recognition, artificial intelligence still makes mistakes no human being would. DARPA’s Next AI Campaign, in essence, is trying to teach computers to think with as much sophistication as human two-year-olds.
Modern AI requires a machine learning algorithm to brute-force its way through tens of thousands of examples until it comes up with statistical rules of thumb. For example, a given picture is probably a cat because 99 percent of images with these particular patterns of pixels were labeled “cat.” By contrast, AI Next aims to develop artificial intelligences can build a conceptual model of how the world works and interpret data based on that model: This picture is probably a cat because it shows two pointy ears, four legs, a tail, and whiskers on a furry body.
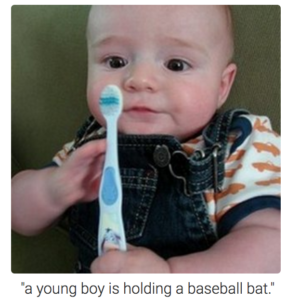
The often-fatal flaw of modern machine learning is that, however many pictures of cats (or tanks or terrorists) you train it on, it still doesn’t have any idea what a “cat” is. It just does lots of math — so much math even the programmers can’t usually understand how it reaches its conclusions — to find clusters of related data (technically, “manifolds”).
Clearly establishing those patterns in the first place requires a tremendous amount of data. An infant AI tries different mathematical permutations largely at random, sees what comes closest to the right answer, the refines those algorithms through trial and error — lots of trial and error: According to the DARPA video at the top of this article, machine learning typically takes some 50,000 to 100,000 pieces of data.
Before you can even start that process, moreover, all those thousands of examples need to be correctly formatted — so the algorithm can process them — and correctly labeled — so the algorithm knows what images are “cats” (or whatever) in the first place. Much of that prep work has to be done by humans, by hand.
China in particular is throwing legions of underpaid workers at the data-labeling problem. As the New York Times reported: “young people go through photos and videos, labeling just about everything they see. That’s a car. That’s a traffic light. That’s bread, that’s milk, that’s chocolate. That’s what it looks like when a person walks.”
Even after all this training, however, what the AI eventually decides is statistically significant may be totally different from what a human would look for, leading to bizarre mistakes. In one experiment, a few well-placed pieces of tape caused a self-driving car AI to mistake a STOP sign for a SPEED LIMIT 45 sign, which would have caused it to speed up instead of stopping — a potentially lethal error.
Machine learning can be deceived by subtle changes in the data: While the AI in this experiment recognized the left-hand image as a panda, distorting it less than 1 percent — in ways imperceptible to the human eye — make it register as an ape.
This is not how a human being learns. When a toddler picks up toys and drops them over and over, they don’t have to try 100,000 times before they figure out what’s happening (though a weary parent might be forgiven for thinking otherwise). The human brain is not building a statistical model of the world, it’s learning a set of rules: “Hey, if I let go of something, and it’s not on top of something else, it falls. Neat!”
That IF-THEN statement is actually a form of algorithm, and so-called first generation artificial intelligence — also known as “expert systems” — is simply a big set of such rules. Tax preparation software, for instance, knows (for example) that IF my income exceeds a certain amount, THEN my deductions don’t count. The problem with this kind of AI is that it can’t learn: Human experts had to write up all the rules in advance so programmers could translate them into machine-readable IF-THEN statements, and if the software came across something that it wasn’t coded for, it didn’t work. AI Next is trying to build software that can — like humans — figure out the rules for itself.
As a side benefit, this rules-based AI should be a lot easier for humans to understand than today’s brute-force number-crunching. Replacing mysterious “black box” artificial intelligences with “explainable AI” is another DARPA priority. The goal, Porter testified, is artificial intelligence that humans can learn to trust.
No comments:
Post a Comment