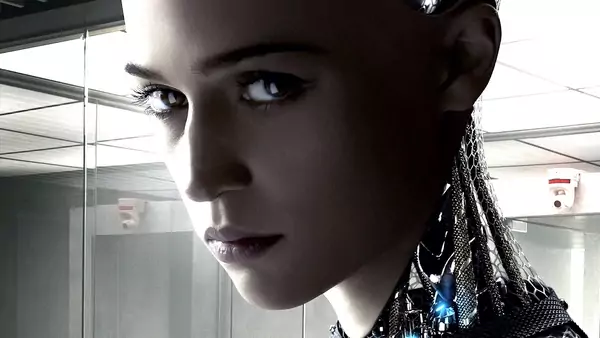
Christopher Zheng is an intern in the Digital and Cyberspace Policy program at the Council on Foreign Relations.
Facebook CEO Mark Zuckerberg and Tesla CEO Elon Musk recently fought over whether artificial intelligence (AI) posed an existential threat to humanity. Musk made the case AI machines could eventually become self-aware and dispose of their human masters, like in the movie Ex Machina, whereas Zuckerberg argued humanity had nothing to fear. It would seem that most of the tech world sides with Zuckerberg given that companies are fast AI applying to fields as diverse as criminal justice and healthcare. With the massive amounts of sensitive data being used by AI to determine the course of individuals’ lives, how vulnerable are AI machines to hackers? There are two ways a malicious actor could own an AI system.
First, machine learning (ML) algorithms—the tools that allow AI to exhibit intelligent behavior—need data to function properly and accurately. While it is possible to make better predictions without ample data, ML algorithms are more accurate with more data. Thus, the primary method for compromising AI so far has been through data manipulation. If data manipulation goes undetected, any organization could struggle to recover the correct data that feeds its AI system, potentially having potentially disastrous consequences in healthcare or finance sectors. In its annual enumeration of threats to the United States, the U.S. intelligence community has highlighted data manipulation as one of its most pressing concerns since 2015.
Second, classification-based ML algorithms work by finding patterns in its data source. Identifying the algorithm’s data source or training method could be a valuable avenue for hackers. For example, computer engineers have created ML algorithms to classify emails as spam by having the algorithms examine a dataset of emails in which some were classified as spam by a human. The algorithms take all words in each email as a data point and establishes patterns that it notices. If “free sample” is in 20 percent of spam emails, the filter learns to classify emails with that phrase as spam. Malicious actors could circumvent this filtration by messing with the algorithm’s ability to equate “free sample” with spam, allowing more emails with “free sample” to bypass the spam filter.
The tech industry knows these problems exist and is trying to fix them. Last month, IBM launched its latest mainframe, IBM Z, and capable of encrypting all data across an entire network. Total encryption of data—made possible by technological advancements in processors— will go a long way in the fight against data manipulation if organizations decide to use it.
Although data manipulation has been the primary method of compromising AI, vulnerabilities will inherently exist in ML code. Even though most companies in the industry already have rigorous procedures for testing and fixing vulnerabilities, software engineers need to be even better about finding and fixing flaws before a software’s public release. Typically, companies release a prototype of its software to a dedicated group of beta-testers to find and fix bugs prior to release. Some companies, like Facebookand Microsoft, are building on this best practice by exploring is the use of AI, in addition to human engineers, to read and reason through thousands of lines of code to quash bugs.
The software industry has also been much more receptive to bug bounty programs to encourage researchers and hackers to report flaws by offering financial rewards. Even the U.S. Justice Department, which has had a contentious relationship with the computer security community in the past, has recently issued guidelines on managing a vulnerability disclosure program within the bounds of the law. However, bounties are not a panacea. Apple learned this the hard way when its payouts for valuable bugs were well below the black market rate.
While industry is committed to investing resources in AI and cybersecurity research, the future of government-funded scientific research under the Trump administration is bleak. Although the administration has proposed increased cyber defense spending, it is slashing research and development funding across multiple agencies and departments. The Trump administration does not seem “worried” about or interested in AI. In its budget proposal, the White House recommended cutting the Department of Homeland Security’s cyber research and development wing and other research-supporting government agencies. Congress, which ultimately holds the purse strings, should recognize more research and development is critical to improving the cybersecurity of AI.
AI is a powerful tool. There’s no perfect solution to stopping data manipulation or eliminating bugs, but a few good ideas exist. Policymakers, technology leaders, and academics need to collaboratively examine how to ensure more widespread use of encryption as well as lobby for continued research funding. Until then, proceed with caution.
No comments:
Post a Comment